Machine Learning for Email Spam Filtering: Revolutionizing Email Security
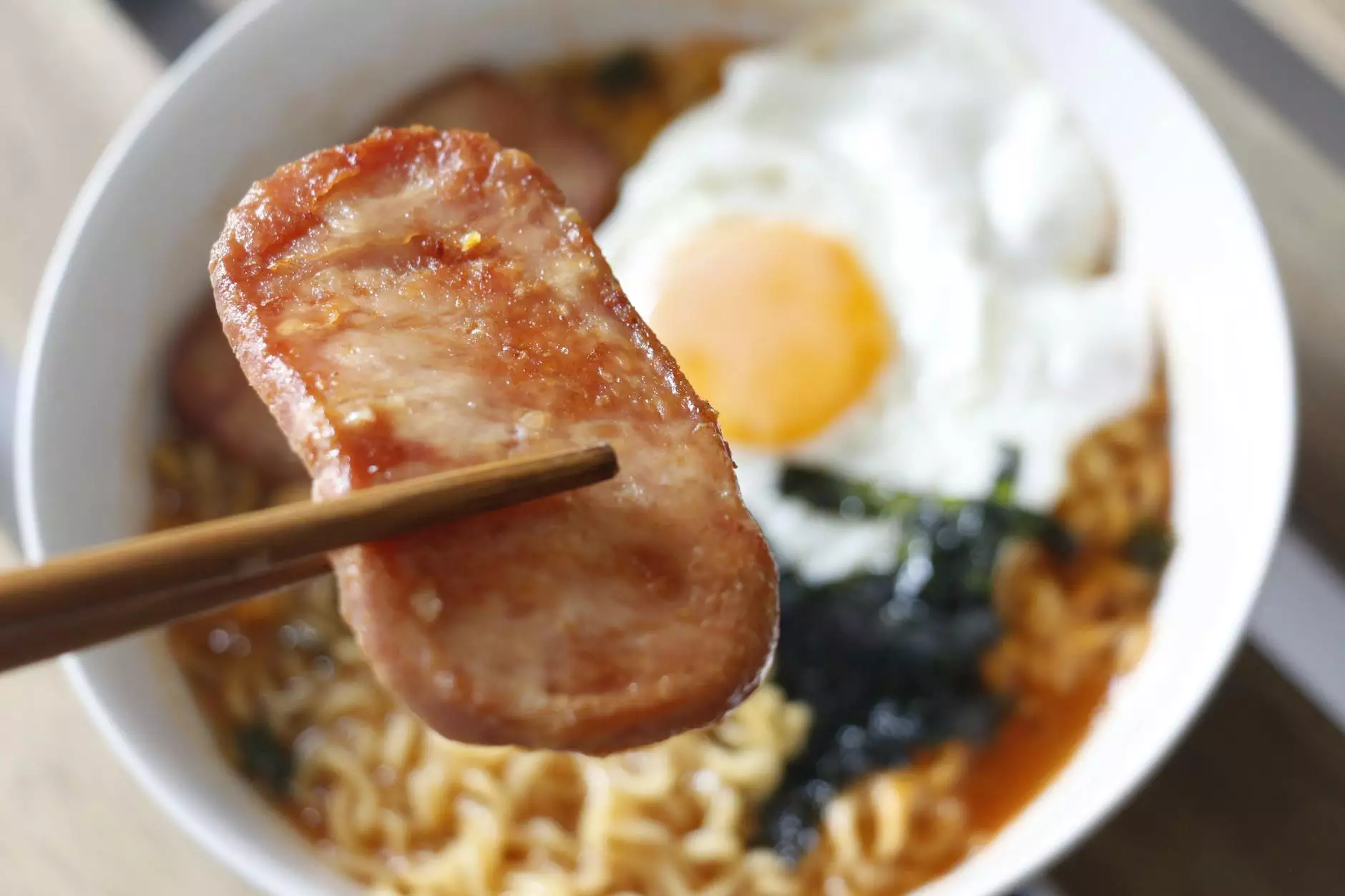
In today's digital landscape, where communication flows rapidly and businesses rely heavily on email, ensuring the safety of email correspondence has never been more critical. Email spam not only clogs inboxes but also poses significant security threats. Enter the realm of machine learning for email spam filtering, a cutting-edge technology that is reshaping the way organizations protect themselves from malicious content. This article will delve deep into the mechanics, advantages, and future perspectives of machine learning in the context of spam filtering.
Understanding Email Spam
Spam emails, often characterized as unsolicited or irrelevant messages, have become a pervasive issue affecting billions of users worldwide. They can vary from benign promotional materials to malicious scams designed to steal personal information. Understanding the nature of these emails is essential in developing effective filter strategies. Here are several types of spam:
- Promotional Spam: Emails advertising products or services.
- Phishing Attacks: Emails trying to obtain sensitive data, like usernames and passwords.
- Malware Spam: Emails that contain attachments or links leading to harmful software.
- Scam Emails: Messages that promise unrealistic financial gain or ask for donations.
The Challenge of Traditional Spam Filtering
Traditional spam filtering techniques relied heavily on rule-based systems and blacklists, where specific keywords or sender addresses would be flagged as spam. While these methods have their merits, they also have significant limitations:
- Inflexibility: Rule-based filters cannot adapt to new, evolving spam techniques.
- High False Positives: Legitimate emails might be incorrectly categorized as spam.
- Manual Updates: Filters require constant updates to maintain effectiveness against newer spam.
Enter Machine Learning
Machine learning represents a paradigm shift in how spam detection is approached. By utilizing sophisticated algorithms and learning from vast datasets, machine learning models can recognize patterns and adapt to new spam strategies, allowing for far more precise filtering.
How Machine Learning for Email Spam Filtering Works
At its core, machine learning for email spam filtering leverages statistical algorithms to identify email characteristics and classify them as either spam or legitimate. The primary steps involved in this process include:
1. Data Collection
To train a machine learning model, a substantial amount of data is required. This data includes examples of both spam and non-spam emails, allowing the algorithm to learn from various characteristics.
2. Feature Extraction
Next, the system extracts relevant features from the emails that can help in identifying their nature. Common features include:
- Content Analysis: The words and phrases used in the email body.
- Sender Reputation: Historical data on the sender’s email address.
- Metadata: Information such as subject lines, timestamps, and email structure.
- Link Analysis: URLs present in the email and their safety status.
3. Training the Model
Using the extracted features, a machine learning model is trained using supervised learning techniques. The model learns to differentiate between spam and legitimate emails based on patterns found in the training data.
4. Continuous Learning
Machine learning models can continuously improve over time as they are exposed to new data. This means they can adapt to new spam tactics and techniques without requiring manual intervention.
The Benefits of Machine Learning in Spam Filtering
Utilizing machine learning for email spam filtering offers several key advantages:
- Higher Accuracy: Machine learning models are often more accurate than traditional filters, reducing the number of false positives.
- Adaptability: The ability to learn from new data allows models to stay ahead of evolving spam strategies.
- Reduced Manual Work: Once trained, machine learning models can automate spam detection, reducing the need for tedious manual updates.
- Enhanced Security: By accurately identifying spam and potential threats, businesses can significantly reduce their risk exposure.
Real-World Applications of Machine Learning in Email Spam Filtering
Numerous organizations have adopted machine learning techniques to enhance their email security protocols. Here are some notable implementations:
1. Google’s Gmail
Google employs advanced machine learning algorithms in Gmail to detect spam emails. Leveraging billions of data points from users, Gmail continuously improves its spam-fighting capabilities, allowing it to filter out up to 99.9% of spam content.
2. Microsoft Outlook
Outlook utilizes machine learning to analyze user behavior and preferences, enabling it to better authenticate messages and filter out unwanted spam while ensuring critical emails reach users.
3. Spambrella
At Spambrella, we integrate cutting-edge machine learning for email spam filtering into our IT Services and Computer Repair offerings. Our system learns from incoming emails, adapting to threats in real-time and providing robust protection against both spam and phishing attacks.
Challenges and Considerations in Machine Learning for Spam Filtering
While machine learning for email spam filtering offers numerous benefits, there are challenges to consider:
- Data Privacy: Organizations must ensure compliance with data protection regulations when collecting email data for training.
- Bias in Data: If the training data is biased, the model may reflect these biases, leading to inefficient spam filtering.
- Computational Resources: Training sophisticated models requires significant computational power and resources.
The Future of Machine Learning in Email Security
As technology continues to advance, the future of machine learning for email spam filtering looks promising. We anticipate the following trends:
- Increased Integration with AI: Combining machine learning with Artificial Intelligence will enable even more sophisticated filtering techniques.
- Enhanced Personalization: Spam filters may become more personalized based on individual user behaviors and preferences, creating a tailored email experience.
- Proactive Threat Detection: Future systems will likely not only identify spam but also predict potential threats before they land in inboxes.
Conclusion
In summary, the application of machine learning for email spam filtering has transformed email security, enabling organizations to defend against the ever-evolving landscape of spam and cyber threats. As businesses like Spambrella continue to innovate and leverage these intelligent systems, the prospect of cleaner, more secure inboxes becomes a reality. By adopting machine learning techniques, companies can enhance their operational efficiency, reduce security risks, and ultimately foster a safer environment for digital communication.
In an era where the potential for cyber threats is omnipresent, investing in advanced email filtering solutions is not just an option; it’s a necessity for safeguarding personal and business information.